#物聯網IoT #人機介面HMI #人工智慧AI #語音交互 #智慧音箱 #人工神經網路ANN #深度神經網路DNN #深度置信網路DBN #遞歸神經網路RNN #卷積神經網路CNN
【語音辨識的沿革】
一直以來,通過語言與機器直接溝通,是很多技術人員追求的目標,可看似簡單的語音交互,卻經歷了長達半個多世紀的技術探索。這期間不管文學還是影視作品,都一次次描繪了人機語音交互的美好場景。但直到 21 世紀初,人與機器的語音交互仍然是令人抓狂的一種操作,遠遠沒有鍵盤和滑鼠來得方便。
在 1952 年,貝爾實驗室研發出了 10 個孤立數位語音的識別系統,為人類的語音辨識開啟了篇章;20 世紀 80 年代開始,以「隱藏式馬可夫模型」(hidden Markov model, HMM) 方法為代表的基於統計模型方法逐漸在語音辨識研究佔據主導地位,能很好地描述語音訊號短時平穩特性,將聲學、語言學、句法等知識集成到統一框架中。此後,HMM 的研究和應用逐漸成為了主流。
李開復在 HMM 模型的基礎上研發出 SPHINX 系統,這是技術人員首次嘗試「非特定人連續語音辨識系統」,其核心框架就是 GMM-HMM 框架,其中 GMM 是指 (Gaussian mixture model,高斯混合模型) 用來對語音的觀察概率進行建模,HMM 則對語音的時序進行建模。同時期發展出的技術,還有 20 世紀 80 年代後期人工神經網路 (ANN) 也成為語音辨識的一個方向。
直至 ANN 後來進化為深度神經網路 (DNN),語音辨識技術才有了本質的突破。到了 20 世紀 90 年代,隨著電腦技術的快速發展,包括個人電腦在內的一大批設備開始嘗試使用語音辨識技術。這一時期劍橋發佈的 HTK 開源工具包大幅降低了語音辨識研究的門檻。然而在接下來的一段時間,GMM-HMM 框架的技術局限性使得其應用效果差強人意。
在語音辨識技術方向中,具有更強的長時建模能力的遞歸神經網路 (又稱:迴圈神經網路,RNN)、卷積神經網路 (CNN),以及在語音辨識領域獨樹一幟的科大訊飛公司提出的 DFCNN 技術相繼出現,進而使得人機語音交互的體驗越來越好,以智慧音箱為代表的語音交互設備受到越來越多的消費者歡迎,比傳統 GMM-HMM 框架性能提升 30~60%,並與 Google 在語音辨識展開深度合作。
延伸閱讀:
《艱難中前行的語音交互技術》
http://www.compotechasia.com/a/feature/2019/0111/40859.html…
(點擊內文標題即可閱讀全文)
#科大訊飛Iflytek #DFCNN
同時也有1部Youtube影片,追蹤數超過16萬的網紅chungdha,也在其Youtube影片中提到,This 2 minute tutorial we are teaching you a quick and easy method to achieve that Miniature/ Diorama / Toy Model / Tilt-Shift Effect in Adobe Premier...
「gaussian model」的推薦目錄:
- 關於gaussian model 在 COMPOTECHAsia電子與電腦 - 陸克文化 Facebook 的最佳貼文
- 關於gaussian model 在 國立陽明交通大學電子工程學系及電子研究所 Facebook 的最讚貼文
- 關於gaussian model 在 chungdha Youtube 的精選貼文
- 關於gaussian model 在 In Depth: Gaussian Mixture Models 的評價
- 關於gaussian model 在 Gaussian Mixture Model with discrete data - Stack Overflow 的評價
- 關於gaussian model 在 What's a component in gaussian mixture model? - Cross ... 的評價
gaussian model 在 國立陽明交通大學電子工程學系及電子研究所 Facebook 的最讚貼文
【課程訊息】8/7(二)「統計訊號處理及通訊導論」由交大電子系 桑梓賢/ 簡鳳村 共同主講,報名網址:http://submic.ee.nctu.edu.tw/curriculum/curriculum.php…
課程大綱:
1.Random Processes
1.1Probability Basics
1.2Definition of a Random Process
1.3Stationary and Wide-Sense Stationary (WSS) Random Processes
1.4WSS Processes in Linear Systems
1.5Power Spectral Density
1.6White Noise Processes
1.7Jointly Gaussian Random Variables
1.8Gaussian Processes
1.9Examples
2.Estimation Theory
2.1Minimum Variance Unbiased Estimator
2.2Cramer-Rao Lower Bound
2.3Sufficient Statistics
2.4Best Linear Unbiased Estimator
2.5Maximum Likelihood Estimator
2.6Moments Estimator
2.7Least Squares Estimator
2.8Minimum Mean Square Error Estimator
2.9Maximum A Posteriori Estimator
3.Detection Theory
3.1Statistical Detection Theory
3.2Neyman-Pearson Theorem
3.3Maximum Likelihood Detector
3.4Maximum Likelihood Detector
3.5Bayes Risk
3.6Composite Hypothesis Testing
4.Basic digital communication
4.1Linear Systems
4.2Signal Space
4.3Modulation
4.4Optimal Receiver
4.5Performance Analysis
4.6Pass-band Systems
4.7Channel Effects and Equalization
5.Information Theory and Channel Coding
5.1Measure of Information
5.2Channel Model and Channel Capacity
5.3Shannon’s Information Theory
5.4Linear Block Codes
5.5Convolutional Codes
5.6Advanced Coding
6.Fading Channels
6.1Channel Characterization
6.2Time Selective Fading
6.3Frequency Selective Fading
6.4Slow/Fast Fading
7.Selected Topics
7.1OFDM
7.2MIMO
gaussian model 在 chungdha Youtube 的精選貼文
This 2 minute tutorial we are teaching you a quick and easy method to achieve that Miniature/ Diorama / Toy Model / Tilt-Shift Effect in Adobe Premiere Pro. For this we easily setup and done with in a single clip using Gaussian Blur and using masking to make this effect possible.
Drone shot filmed with:
DJI Mavic Pro - https://amzn.to/2UDvmPm
Check out my Adobe Premiere Pro Effects tutorials here:
https://www.youtube.com/playlist?list=PLAdjMWVKvc3B2SqrDQB-r5EMxyrn29h6z
Edited with Adobe Premiere Pro - http://goo.gl/k2EagF
#tiltshift #premierepro #chungdha
If you appreciate what I do, you can support me by donating any amount here on paypal:
http://paypal.me/ChungDha
? Facebook: http://www.facebook.com/chungdha
? Website: http://www.chungdha.nl
? Instagram: https://www.instagram.com/chungdha/
? Twitter: https://twitter.com/chungdha
For any Questions Please Join our Facebook Group: https://www.facebook.com/groups/chungdhagroup
Business Inquiries, Sponsors & Collaboration email contact@chungdha.com
Chung Dha © 2020 Tsuen Wan, Hong Kong
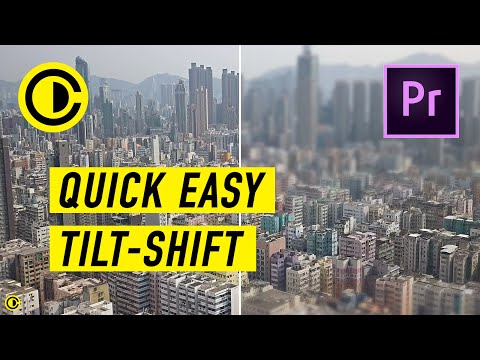
gaussian model 在 What's a component in gaussian mixture model? - Cross ... 的推薦與評價
A mixture of Gaussians is defined as a linear combination of multiple Gaussian distributions. Thus it has multiple modes. ... <看更多>
gaussian model 在 In Depth: Gaussian Mixture Models 的推薦與評價
Here the mixture of 16 Gaussians serves not to find separated clusters of data, but rather to model the overall distribution of the input data. This is a ... ... <看更多>